To understand how contemplative programming influences thinking, emotions, and perceptions, we must peek into the mind. Current assessments primarily rely on self-report. Self-report surveys are efficient but require the respondent to evaluate their own consciousness, a challenging process that can also introduce bias. This issue can be eliminated by asking respondents to recall their experiences and having experts objectively evaluate those recollections. Machine learning provides an avenue for efficient, objective coding of respondents’ recall to address the resource costs of manual coding. This is the approach of the Objective Awareness and Mindfulness Measure (OAMM). With this project, we will develop the OAMM for teachers. In addition to serving as a first variant, the OAMM for teachers will provide a much-needed tool to better understand how teacher mindfulness programming translates into beneficial teacher and student outcomes. For this project, we will collect written introspective recall of classroom interactions from 432 teachers. Eight diverse contemplative experts with K-12 experience will score these interactions for evidence of mindfulness (e.g., present-focused orientation, empathy, nonjudgment, self-compassion). These expert scores will be used to train a computer to directly score future teacher recall of classroom interactions, creating a new and efficient assessment of mindfulness.
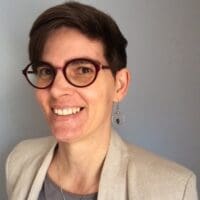
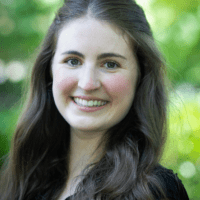