Compassion, one of the immeasurables, is the genuine wish that suffering and hardship of sentient beings be alleviated. Although it is a quality of personal well-being, it is not cultivated in isolation. It exemplifies the interpersonal because it is a willingness to accompany pain and fragility of self/others in intimate and balanced ways. Compassion naturally arises from wholeheartedly investigating pain. Internal Family System (IFS) is a two-stage, structured contemplative practice enabling the discovery of a naturally, pre-existing compassionate witness through a functional investigation of the mind’s “parts.’ ‘Parts,’ are viewed as clusters of motivations, sensations, thoughts, emotions, and perceptions – shaped by developmental, social, and cultural contexts. Distinct from parts, one’s core consciousness is suffused with curiosity, loving-kindness, compassion, equanimity and joy. The aim of this study is to explore the feasibility of deep learning to generate text-based digital biomarker correlates of inner compassion. By analyzing 114 hours of video footage from three recently completed research trials of a multi week IFS-based intervention, we aim to find dynamic digital signatures of inner compassion within a social context. Multimodal digital biomarkers provide non-intrusive, scalable, and quantitative ways for understanding how different individuals may respond to contemplative practices.
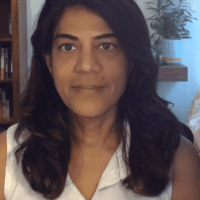